Understanding Well-Being in the Face of Large-Scale Crisis using Advanced Machine Learning Methods
- taliamst
- Mar 9, 2022
- 1 min read
In this study, we based our analyses on SPI's 4-wave comprehensive households data to reveal multileveled risk and protective factors to wellbeing during the COVID-19 pandemic.
Dr. Talia M. Schwartz-Tayri, Ph.D
Head and founder, the AI for SW lab
Spitzer Department of Social Work at Ben Gurion University of the Negev
Dr. Michael Fire, Ph.D
Founder of the Data Science for Social Good Lab
Software and Information Systems Engineering Department at Ben Gurion University of the Negev
Guy Navon
Software and Information Systems Engineering Department at Ben Gurion University of the Negev
Dr. Michal Grinstein-Weiss, Ph.D., Shanti K Khinduka Distinguished Professor
Washington University in St Louis, Saint Louis, MO
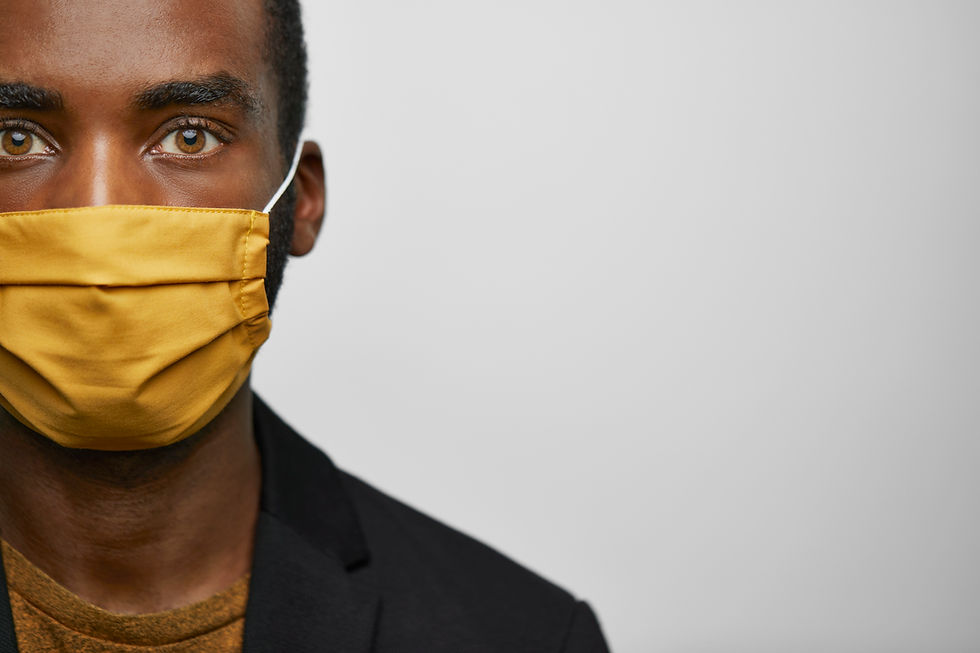
We based our analyses on SPI's 4-wave comprehensive households data to reveal multileveled risk and protective factors to wellbeing during the COVID-19 pandemic.
This project used an innovative machine learning approach to expose multileveled factors to explain resiliency during the COVID-19 pandemic and follows individuals' wellbeing changes throughout a year and a half.
Although recent resiliency research was groundbreaking, theoretical models have not incorporated the unique effects of multileveled factors, including the government policy, on individual and household resiliency.
To address this gap, we used SPI novel longitudinal 4-wave dataset (N=2,363) to reveal personal, familial, and policy-level features with the strongest predictive power on wellbeing. Data included comprehensive information on individual's and household sociodemographic characteristics, psychological wellbeing, emotional support, generalized anxiety, income loss due to COVID-19, nutrition insecurity, financial assets, government benefits, and more.
Comments